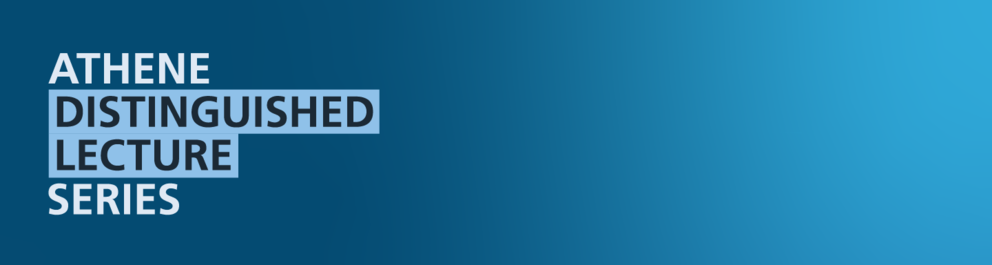
2. Juli 2024 | 14:00 - 15:00 Uhr: Tal Shapira, Co-Founder & CTO at Reco
Die Lecture wird hybrid durchgeführt.
Veranstaltungsort für die Präsenzveranstaltung ist:
TU Darmstadt | Altes Hauptgebäude (S1|03) | Wilhelm-Köhler-Saal (Raum 283) | Hochschulstraße 1 | 64289 Darmstadt
Online wird das Event über MS Teams durchgeführt.
Bei einer Präsenzteilnahme besteht die Möglichkeit, sich nach dem Vortrag persönlich mit den wichtigsten Akteuren der Cybersicherheit zu treffen und auszutauschen.
Biography
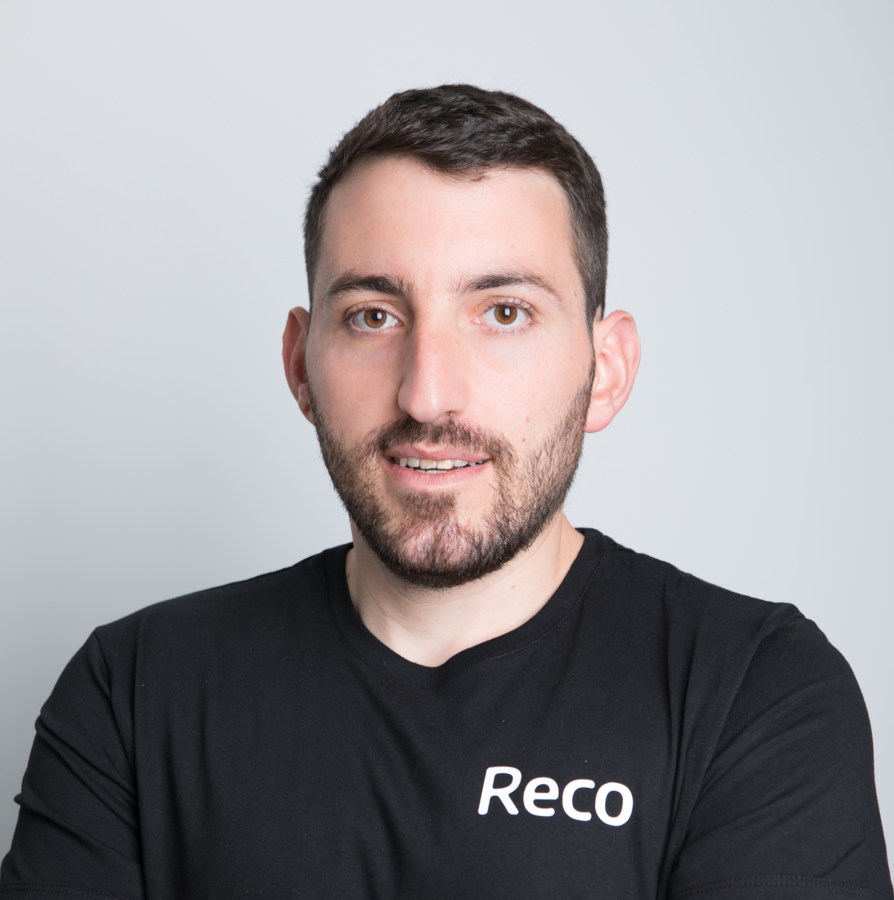
Tal Shapira, Ph.D., conducting research in the fields of deep learning, computer networks, and
cybersecurity. Currently a Post-Doc at the School of Computer Science, The Hebrew University of
Jerusalem, advised by Prof. Anat Bremler-Barr. Tal completed a Post-Doc at Reichman University, and
graduated magna cum laude with a P.hD. from Tel-Aviv University, where he was advised by Prof. Yuval
Shavitt.
Tal is the Co-Founder & CTO at Reco, which develops a SaaS Security platform, and a former head of a
cybersecurity R&D group within the Israeli Prime Minister’s Office.
A Deep Learning Approach for Detecting IP Hijack Attacks
Abstract
In recent years, there have been many reports of BGP Prefix hijacking of nations and large companies, as
more than 40% of the network operators reported that their organization had been a victim of a hijack in
the past. BGP hijack attacks deflect traffic between endpoints through the attacker network, leading to
man-in-the-middle attacks.
In this talk, we will discuss a deep learning approach for detecting IP hijack attacks on the internet. To
detect these attacks, we propose a system that harnesses deep learning techniques. First, we create a
dense vector representation of Autonomous Systems (ASes) using BGP routing update messages, called
BGP2Vec. This representation allows us to identify the type of relationship between ASes, known as ToR,
and detect hijack attacks using valley-free routing rules. Additionally, we train a model using complete
routes to identify hijacked routes, taking into account small deviations from valley-free routing. To
improve the system's ability to identify the cause of a flagged route, we also propose a Source-Aware Self-
Attention (SASA) layer. Lastly, we introduce a novel approach, called AP2Vec, that detects functional
changes in ASes during a hijack attack by comparing the embedding of a new route to the embedding of
old routes. We demonstrate that our approach strikes the best balance between a high detection rate and
a low number of flagged events.